MovieLens#
Movie Lens is a website that helps users find the movies they like and where they will rate the recommended movies. MovieLens 1M dataset is a dataset including the observations collected in an online movie recommendation experiment and is widely used to generate data for online bandit simulation studies. The goal of the simulation studies below is to learn the reward distribution of different movie genres and hence to recommend the optimal movie genres to the users to optimize the cumulative user satisfaction. In other words, every time a user visits the website, the agent will recommend a movie genre (\(A_t\)) to the user, and then the user will give a rating (\(R_t\)) to the genre recommended. We assume that users’ satisfaction is fully reflected through the ratings. Therefore, the ultimate goal of the bandit algorithms is to optimize the cumulative ratings received by finding and recommending the optimal movie genre that will receive the highest rating. In this tutorial, we mainly focus on the following 4 Genres, including
Comedy: \(a=0\),
Drama: \(a=1\)
Action: \(a=2\),
Thriller: \(a=3\),
Sci-Fi: \(a=4\).
Therefore, \(K=5\). For each user, feature information, including age, gender and occupation, are available:
age: numerical, from 18 to 56,
gender: binary, =1 if male,
college/grad student: binary, =1 if a college/grad student,
executive/managerial: binary, =1 if a executive/managerial,
technician/engineer: binary, =1 if a technician/engineer,
other: binary, =1 if having other occupations other than the rest of the four occupations,
academic/educator: if an academic/educator, then all the previous occupation-related variables = 0 (baseline).
The realized reward \(R_t\) is a numerical variable, taking the value of \(\{1,2,3,4,5\}\),with 1 being the least satisfied and 5 being the most satisfied. In the following, we first perform causal effect learning on the logged data and output the estimated reward for each movie genre. Then, we conduct online learning to efficiently explore the optimal policy utilizing both the estimation results and new information collected through real-time online iteraction.
Causal Effect Learning#
import pandas as pd
import numpy as np
from sklearn.linear_model import LinearRegression
from causaldm.learners.CPL4.CMAB import _env_realCMAB as _env
from causaldm.learners.CPL4.CMAB import LinTS
import warnings
warnings.filterwarnings('ignore')
env = _env.Single_Contextual_Env(seed = 0, Binary = False)
logged_data, arms = env.get_logged_dat()
WARNING (theano.tensor.blas): Using NumPy C-API based implementation for BLAS functions.
logged_data.keys()
dict_keys(['Comedy', 'Drama', 'Action', 'Thriller', 'Sci-Fi'])
logged_data['Comedy']
user_id | movie_id | rating | age | Comedy | Drama | Action | Thriller | Sci-Fi | gender_M | occupation_academic/educator | occupation_college/grad student | occupation_executive/managerial | occupation_other | occupation_technician/engineer | |
---|---|---|---|---|---|---|---|---|---|---|---|---|---|---|---|
685706 | 48 | 2042.0 | 2.0 | 25.0 | 1.0 | 0.0 | 0.0 | 0.0 | 0.0 | 1.0 | 0.0 | 1.0 | 0.0 | 0.0 | 0.0 |
499683 | 48 | 2424.0 | 4.0 | 25.0 | 1.0 | 0.0 | 0.0 | 0.0 | 0.0 | 1.0 | 0.0 | 1.0 | 0.0 | 0.0 | 0.0 |
694860 | 53 | 330.0 | 3.0 | 25.0 | 1.0 | 0.0 | 0.0 | 0.0 | 0.0 | 1.0 | 0.0 | 0.0 | 0.0 | 1.0 | 0.0 |
207691 | 169 | 3671.0 | 4.0 | 25.0 | 1.0 | 0.0 | 0.0 | 0.0 | 0.0 | 1.0 | 0.0 | 0.0 | 1.0 | 0.0 | 0.0 |
425839 | 169 | 3869.0 | 3.0 | 25.0 | 1.0 | 0.0 | 0.0 | 0.0 | 0.0 | 1.0 | 0.0 | 0.0 | 1.0 | 0.0 | 0.0 |
... | ... | ... | ... | ... | ... | ... | ... | ... | ... | ... | ... | ... | ... | ... | ... |
213638 | 4140.0 | 153.0 | 2.0 | 25.0 | 1.0 | 0.0 | 0.0 | 0.0 | 0.0 | 1.0 | 0.0 | 0.0 | 0.0 | 1.0 | 0.0 |
242271 | 4140.0 | 435.0 | 1.0 | 25.0 | 1.0 | 0.0 | 0.0 | 0.0 | 0.0 | 1.0 | 0.0 | 0.0 | 0.0 | 1.0 | 0.0 |
717322 | 4411 | 910.0 | 5.0 | 18.0 | 1.0 | 0.0 | 0.0 | 0.0 | 0.0 | 1.0 | 0.0 | 1.0 | 0.0 | 0.0 | 0.0 |
345927 | 4411.0 | 45.0 | 2.0 | 18.0 | 1.0 | 0.0 | 0.0 | 0.0 | 0.0 | 1.0 | 0.0 | 1.0 | 0.0 | 0.0 | 0.0 |
870113 | 5878 | 1226.0 | 5.0 | 25.0 | 1.0 | 0.0 | 0.0 | 0.0 | 0.0 | 0.0 | 0.0 | 0.0 | 0.0 | 1.0 | 0.0 |
406 rows × 15 columns
userinfo_index = np.array([3,9,11,12,13,14])
movie_generes = ['Comedy', 'Drama', 'Action', 'Thriller', 'Sci-Fi']
# convert the sampled dataset of interest to dataframe format
data_CEL_sample = logged_data['Comedy']
for movie_genere in movie_generes[1:5]:
data_CEL_sample = pd.concat([data_CEL_sample, logged_data[movie_genere]])
len(data_CEL_sample) # the total sample size we selected: n=1286
1286
nonlinear model fitting#
models_CEL = {}
# initialize the models we'll fit in Causal Effect Learning
for i in movie_generes:
models_CEL[i] = None
from lightgbm import LGBMRegressor
for movie_genere in movie_generes:
models_CEL[movie_genere] = LGBMRegressor(max_depth=3)
models_CEL[movie_genere].fit(data_CEL_sample.iloc[np.where(data_CEL_sample[movie_genere]==1)[0],userinfo_index],data_CEL_sample.iloc[np.where(data_CEL_sample[movie_genere]==1)[0],2] )
[LightGBM] [Warning] Found whitespace in feature_names, replace with underlines
[LightGBM] [Info] Auto-choosing row-wise multi-threading, the overhead of testing was 0.000031 seconds.
You can set `force_row_wise=true` to remove the overhead.
And if memory is not enough, you can set `force_col_wise=true`.
[LightGBM] [Info] Total Bins 18
[LightGBM] [Info] Number of data points in the train set: 406, number of used features: 6
[LightGBM] [Info] Start training from score 3.339901
[LightGBM] [Warning] No further splits with positive gain, best gain: -inf
[LightGBM] [Warning] No further splits with positive gain, best gain: -inf
[LightGBM] [Warning] No further splits with positive gain, best gain: -inf
[LightGBM] [Warning] No further splits with positive gain, best gain: -inf
[LightGBM] [Warning] No further splits with positive gain, best gain: -inf
[LightGBM] [Warning] No further splits with positive gain, best gain: -inf
[LightGBM] [Warning] No further splits with positive gain, best gain: -inf
[LightGBM] [Warning] No further splits with positive gain, best gain: -inf
[LightGBM] [Warning] No further splits with positive gain, best gain: -inf
[LightGBM] [Warning] No further splits with positive gain, best gain: -inf
[LightGBM] [Warning] No further splits with positive gain, best gain: -inf
[LightGBM] [Warning] No further splits with positive gain, best gain: -inf
[LightGBM] [Warning] No further splits with positive gain, best gain: -inf
[LightGBM] [Warning] No further splits with positive gain, best gain: -inf
[LightGBM] [Warning] No further splits with positive gain, best gain: -inf
[LightGBM] [Warning] No further splits with positive gain, best gain: -inf
[LightGBM] [Warning] No further splits with positive gain, best gain: -inf
[LightGBM] [Warning] No further splits with positive gain, best gain: -inf
[LightGBM] [Warning] No further splits with positive gain, best gain: -inf
[LightGBM] [Warning] No further splits with positive gain, best gain: -inf
[LightGBM] [Warning] No further splits with positive gain, best gain: -inf
[LightGBM] [Warning] No further splits with positive gain, best gain: -inf
[LightGBM] [Warning] No further splits with positive gain, best gain: -inf
[LightGBM] [Warning] No further splits with positive gain, best gain: -inf
[LightGBM] [Warning] No further splits with positive gain, best gain: -inf
[LightGBM] [Warning] No further splits with positive gain, best gain: -inf
[LightGBM] [Warning] No further splits with positive gain, best gain: -inf
[LightGBM] [Warning] No further splits with positive gain, best gain: -inf
[LightGBM] [Warning] No further splits with positive gain, best gain: -inf
[LightGBM] [Warning] No further splits with positive gain, best gain: -inf
[LightGBM] [Warning] No further splits with positive gain, best gain: -inf
[LightGBM] [Warning] No further splits with positive gain, best gain: -inf
[LightGBM] [Warning] No further splits with positive gain, best gain: -inf
[LightGBM] [Warning] No further splits with positive gain, best gain: -inf
[LightGBM] [Warning] No further splits with positive gain, best gain: -inf
[LightGBM] [Warning] No further splits with positive gain, best gain: -inf
[LightGBM] [Warning] No further splits with positive gain, best gain: -inf
[LightGBM] [Warning] No further splits with positive gain, best gain: -inf
[LightGBM] [Warning] No further splits with positive gain, best gain: -inf
[LightGBM] [Warning] No further splits with positive gain, best gain: -inf
[LightGBM] [Warning] No further splits with positive gain, best gain: -inf
[LightGBM] [Warning] No further splits with positive gain, best gain: -inf
[LightGBM] [Warning] No further splits with positive gain, best gain: -inf
[LightGBM] [Warning] No further splits with positive gain, best gain: -inf
[LightGBM] [Warning] No further splits with positive gain, best gain: -inf
[LightGBM] [Warning] No further splits with positive gain, best gain: -inf
[LightGBM] [Warning] No further splits with positive gain, best gain: -inf
[LightGBM] [Warning] No further splits with positive gain, best gain: -inf
[LightGBM] [Warning] No further splits with positive gain, best gain: -inf
[LightGBM] [Warning] No further splits with positive gain, best gain: -inf
[LightGBM] [Warning] No further splits with positive gain, best gain: -inf
[LightGBM] [Warning] No further splits with positive gain, best gain: -inf
[LightGBM] [Warning] No further splits with positive gain, best gain: -inf
[LightGBM] [Warning] No further splits with positive gain, best gain: -inf
[LightGBM] [Warning] No further splits with positive gain, best gain: -inf
[LightGBM] [Warning] No further splits with positive gain, best gain: -inf
[LightGBM] [Warning] No further splits with positive gain, best gain: -inf
[LightGBM] [Warning] No further splits with positive gain, best gain: -inf
[LightGBM] [Warning] No further splits with positive gain, best gain: -inf
[LightGBM] [Warning] No further splits with positive gain, best gain: -inf
[LightGBM] [Warning] No further splits with positive gain, best gain: -inf
[LightGBM] [Warning] No further splits with positive gain, best gain: -inf
[LightGBM] [Warning] No further splits with positive gain, best gain: -inf
[LightGBM] [Warning] No further splits with positive gain, best gain: -inf
[LightGBM] [Warning] No further splits with positive gain, best gain: -inf
[LightGBM] [Warning] No further splits with positive gain, best gain: -inf
[LightGBM] [Warning] No further splits with positive gain, best gain: -inf
[LightGBM] [Warning] No further splits with positive gain, best gain: -inf
[LightGBM] [Warning] No further splits with positive gain, best gain: -inf
[LightGBM] [Warning] No further splits with positive gain, best gain: -inf
[LightGBM] [Warning] No further splits with positive gain, best gain: -inf
[LightGBM] [Warning] No further splits with positive gain, best gain: -inf
[LightGBM] [Warning] No further splits with positive gain, best gain: -inf
[LightGBM] [Warning] No further splits with positive gain, best gain: -inf
[LightGBM] [Warning] No further splits with positive gain, best gain: -inf
[LightGBM] [Warning] No further splits with positive gain, best gain: -inf
[LightGBM] [Warning] No further splits with positive gain, best gain: -inf
[LightGBM] [Warning] No further splits with positive gain, best gain: -inf
[LightGBM] [Warning] No further splits with positive gain, best gain: -inf
[LightGBM] [Warning] No further splits with positive gain, best gain: -inf
[LightGBM] [Warning] No further splits with positive gain, best gain: -inf
[LightGBM] [Warning] No further splits with positive gain, best gain: -inf
[LightGBM] [Warning] No further splits with positive gain, best gain: -inf
[LightGBM] [Warning] No further splits with positive gain, best gain: -inf
[LightGBM] [Warning] No further splits with positive gain, best gain: -inf
[LightGBM] [Warning] No further splits with positive gain, best gain: -inf
[LightGBM] [Warning] No further splits with positive gain, best gain: -inf
[LightGBM] [Warning] No further splits with positive gain, best gain: -inf
[LightGBM] [Warning] No further splits with positive gain, best gain: -inf
[LightGBM] [Warning] No further splits with positive gain, best gain: -inf
[LightGBM] [Warning] No further splits with positive gain, best gain: -inf
[LightGBM] [Warning] No further splits with positive gain, best gain: -inf
[LightGBM] [Warning] No further splits with positive gain, best gain: -inf
[LightGBM] [Warning] No further splits with positive gain, best gain: -inf
[LightGBM] [Warning] No further splits with positive gain, best gain: -inf
[LightGBM] [Warning] No further splits with positive gain, best gain: -inf
[LightGBM] [Warning] No further splits with positive gain, best gain: -inf
[LightGBM] [Warning] No further splits with positive gain, best gain: -inf
[LightGBM] [Warning] No further splits with positive gain, best gain: -inf
[LightGBM] [Warning] No further splits with positive gain, best gain: -inf
[LightGBM] [Warning] Found whitespace in feature_names, replace with underlines
[LightGBM] [Info] Auto-choosing col-wise multi-threading, the overhead of testing was 0.000049 seconds.
You can set `force_col_wise=true` to remove the overhead.
[LightGBM] [Info] Total Bins 18
[LightGBM] [Info] Number of data points in the train set: 393, number of used features: 6
[LightGBM] [Info] Start training from score 3.483461
[LightGBM] [Warning] No further splits with positive gain, best gain: -inf
[LightGBM] [Warning] No further splits with positive gain, best gain: -inf
[LightGBM] [Warning] No further splits with positive gain, best gain: -inf
[LightGBM] [Warning] No further splits with positive gain, best gain: -inf
[LightGBM] [Warning] No further splits with positive gain, best gain: -inf
[LightGBM] [Warning] No further splits with positive gain, best gain: -inf
[LightGBM] [Warning] No further splits with positive gain, best gain: -inf
[LightGBM] [Warning] No further splits with positive gain, best gain: -inf
[LightGBM] [Warning] No further splits with positive gain, best gain: -inf
[LightGBM] [Warning] No further splits with positive gain, best gain: -inf
[LightGBM] [Warning] No further splits with positive gain, best gain: -inf
[LightGBM] [Warning] No further splits with positive gain, best gain: -inf
[LightGBM] [Warning] No further splits with positive gain, best gain: -inf
[LightGBM] [Warning] No further splits with positive gain, best gain: -inf
[LightGBM] [Warning] No further splits with positive gain, best gain: -inf
[LightGBM] [Warning] No further splits with positive gain, best gain: -inf
[LightGBM] [Warning] No further splits with positive gain, best gain: -inf
[LightGBM] [Warning] No further splits with positive gain, best gain: -inf
[LightGBM] [Warning] No further splits with positive gain, best gain: -inf
[LightGBM] [Warning] No further splits with positive gain, best gain: -inf
[LightGBM] [Warning] No further splits with positive gain, best gain: -inf
[LightGBM] [Warning] No further splits with positive gain, best gain: -inf
[LightGBM] [Warning] No further splits with positive gain, best gain: -inf
[LightGBM] [Warning] No further splits with positive gain, best gain: -inf
[LightGBM] [Warning] No further splits with positive gain, best gain: -inf
[LightGBM] [Warning] No further splits with positive gain, best gain: -inf
[LightGBM] [Warning] No further splits with positive gain, best gain: -inf
[LightGBM] [Warning] No further splits with positive gain, best gain: -inf
[LightGBM] [Warning] No further splits with positive gain, best gain: -inf
[LightGBM] [Warning] No further splits with positive gain, best gain: -inf
[LightGBM] [Warning] No further splits with positive gain, best gain: -inf
[LightGBM] [Warning] No further splits with positive gain, best gain: -inf
[LightGBM] [Warning] No further splits with positive gain, best gain: -inf
[LightGBM] [Warning] No further splits with positive gain, best gain: -inf
[LightGBM] [Warning] No further splits with positive gain, best gain: -inf
[LightGBM] [Warning] No further splits with positive gain, best gain: -inf
[LightGBM] [Warning] No further splits with positive gain, best gain: -inf
[LightGBM] [Warning] No further splits with positive gain, best gain: -inf
[LightGBM] [Warning] No further splits with positive gain, best gain: -inf
[LightGBM] [Warning] No further splits with positive gain, best gain: -inf
[LightGBM] [Warning] No further splits with positive gain, best gain: -inf
[LightGBM] [Warning] No further splits with positive gain, best gain: -inf
[LightGBM] [Warning] No further splits with positive gain, best gain: -inf
[LightGBM] [Warning] No further splits with positive gain, best gain: -inf
[LightGBM] [Warning] No further splits with positive gain, best gain: -inf
[LightGBM] [Warning] No further splits with positive gain, best gain: -inf
[LightGBM] [Warning] No further splits with positive gain, best gain: -inf
[LightGBM] [Warning] No further splits with positive gain, best gain: -inf
[LightGBM] [Warning] No further splits with positive gain, best gain: -inf
[LightGBM] [Warning] No further splits with positive gain, best gain: -inf
[LightGBM] [Warning] No further splits with positive gain, best gain: -inf
[LightGBM] [Warning] No further splits with positive gain, best gain: -inf
[LightGBM] [Warning] No further splits with positive gain, best gain: -inf
[LightGBM] [Warning] No further splits with positive gain, best gain: -inf
[LightGBM] [Warning] No further splits with positive gain, best gain: -inf
[LightGBM] [Warning] No further splits with positive gain, best gain: -inf
[LightGBM] [Warning] No further splits with positive gain, best gain: -inf
[LightGBM] [Warning] No further splits with positive gain, best gain: -inf
[LightGBM] [Warning] No further splits with positive gain, best gain: -inf
[LightGBM] [Warning] No further splits with positive gain, best gain: -inf
[LightGBM] [Warning] No further splits with positive gain, best gain: -inf
[LightGBM] [Warning] No further splits with positive gain, best gain: -inf
[LightGBM] [Warning] No further splits with positive gain, best gain: -inf
[LightGBM] [Warning] No further splits with positive gain, best gain: -inf
[LightGBM] [Warning] No further splits with positive gain, best gain: -inf
[LightGBM] [Warning] No further splits with positive gain, best gain: -inf
[LightGBM] [Warning] No further splits with positive gain, best gain: -inf
[LightGBM] [Warning] No further splits with positive gain, best gain: -inf
[LightGBM] [Warning] No further splits with positive gain, best gain: -inf
[LightGBM] [Warning] No further splits with positive gain, best gain: -inf
[LightGBM] [Warning] No further splits with positive gain, best gain: -inf
[LightGBM] [Warning] No further splits with positive gain, best gain: -inf
[LightGBM] [Warning] No further splits with positive gain, best gain: -inf
[LightGBM] [Warning] No further splits with positive gain, best gain: -inf
[LightGBM] [Warning] No further splits with positive gain, best gain: -inf
[LightGBM] [Warning] No further splits with positive gain, best gain: -inf
[LightGBM] [Warning] No further splits with positive gain, best gain: -inf
[LightGBM] [Warning] No further splits with positive gain, best gain: -inf
[LightGBM] [Warning] No further splits with positive gain, best gain: -inf
[LightGBM] [Warning] No further splits with positive gain, best gain: -inf
[LightGBM] [Warning] No further splits with positive gain, best gain: -inf
[LightGBM] [Warning] No further splits with positive gain, best gain: -inf
[LightGBM] [Warning] No further splits with positive gain, best gain: -inf
[LightGBM] [Warning] No further splits with positive gain, best gain: -inf
[LightGBM] [Warning] No further splits with positive gain, best gain: -inf
[LightGBM] [Warning] No further splits with positive gain, best gain: -inf
[LightGBM] [Warning] No further splits with positive gain, best gain: -inf
[LightGBM] [Warning] No further splits with positive gain, best gain: -inf
[LightGBM] [Warning] No further splits with positive gain, best gain: -inf
[LightGBM] [Warning] No further splits with positive gain, best gain: -inf
[LightGBM] [Warning] No further splits with positive gain, best gain: -inf
[LightGBM] [Warning] No further splits with positive gain, best gain: -inf
[LightGBM] [Warning] No further splits with positive gain, best gain: -inf
[LightGBM] [Warning] No further splits with positive gain, best gain: -inf
[LightGBM] [Warning] No further splits with positive gain, best gain: -inf
[LightGBM] [Warning] No further splits with positive gain, best gain: -inf
[LightGBM] [Warning] No further splits with positive gain, best gain: -inf
[LightGBM] [Warning] No further splits with positive gain, best gain: -inf
[LightGBM] [Warning] No further splits with positive gain, best gain: -inf
[LightGBM] [Warning] No further splits with positive gain, best gain: -inf
[LightGBM] [Warning] Found whitespace in feature_names, replace with underlines
[LightGBM] [Info] Auto-choosing col-wise multi-threading, the overhead of testing was 0.000048 seconds.
You can set `force_col_wise=true` to remove the overhead.
[LightGBM] [Info] Total Bins 17
[LightGBM] [Info] Number of data points in the train set: 235, number of used features: 6
[LightGBM] [Info] Start training from score 3.319149
[LightGBM] [Warning] No further splits with positive gain, best gain: -inf
[LightGBM] [Warning] No further splits with positive gain, best gain: -inf
[LightGBM] [Warning] No further splits with positive gain, best gain: -inf
[LightGBM] [Warning] No further splits with positive gain, best gain: -inf
[LightGBM] [Warning] No further splits with positive gain, best gain: -inf
[LightGBM] [Warning] No further splits with positive gain, best gain: -inf
[LightGBM] [Warning] No further splits with positive gain, best gain: -inf
[LightGBM] [Warning] No further splits with positive gain, best gain: -inf
[LightGBM] [Warning] No further splits with positive gain, best gain: -inf
[LightGBM] [Warning] No further splits with positive gain, best gain: -inf
[LightGBM] [Warning] No further splits with positive gain, best gain: -inf
[LightGBM] [Warning] No further splits with positive gain, best gain: -inf
[LightGBM] [Warning] No further splits with positive gain, best gain: -inf
[LightGBM] [Warning] No further splits with positive gain, best gain: -inf
[LightGBM] [Warning] No further splits with positive gain, best gain: -inf
[LightGBM] [Warning] No further splits with positive gain, best gain: -inf
[LightGBM] [Warning] No further splits with positive gain, best gain: -inf
[LightGBM] [Warning] No further splits with positive gain, best gain: -inf
[LightGBM] [Warning] No further splits with positive gain, best gain: -inf
[LightGBM] [Warning] No further splits with positive gain, best gain: -inf
[LightGBM] [Warning] No further splits with positive gain, best gain: -inf
[LightGBM] [Warning] No further splits with positive gain, best gain: -inf
[LightGBM] [Warning] No further splits with positive gain, best gain: -inf
[LightGBM] [Warning] No further splits with positive gain, best gain: -inf
[LightGBM] [Warning] No further splits with positive gain, best gain: -inf
[LightGBM] [Warning] No further splits with positive gain, best gain: -inf
[LightGBM] [Warning] No further splits with positive gain, best gain: -inf
[LightGBM] [Warning] No further splits with positive gain, best gain: -inf
[LightGBM] [Warning] No further splits with positive gain, best gain: -inf
[LightGBM] [Warning] No further splits with positive gain, best gain: -inf
[LightGBM] [Warning] No further splits with positive gain, best gain: -inf
[LightGBM] [Warning] No further splits with positive gain, best gain: -inf
[LightGBM] [Warning] No further splits with positive gain, best gain: -inf
[LightGBM] [Warning] No further splits with positive gain, best gain: -inf
[LightGBM] [Warning] No further splits with positive gain, best gain: -inf
[LightGBM] [Warning] No further splits with positive gain, best gain: -inf
[LightGBM] [Warning] No further splits with positive gain, best gain: -inf
[LightGBM] [Warning] No further splits with positive gain, best gain: -inf
[LightGBM] [Warning] No further splits with positive gain, best gain: -inf
[LightGBM] [Warning] No further splits with positive gain, best gain: -inf
[LightGBM] [Warning] No further splits with positive gain, best gain: -inf
[LightGBM] [Warning] No further splits with positive gain, best gain: -inf
[LightGBM] [Warning] No further splits with positive gain, best gain: -inf
[LightGBM] [Warning] No further splits with positive gain, best gain: -inf
[LightGBM] [Warning] No further splits with positive gain, best gain: -inf
[LightGBM] [Warning] No further splits with positive gain, best gain: -inf
[LightGBM] [Warning] No further splits with positive gain, best gain: -inf
[LightGBM] [Warning] No further splits with positive gain, best gain: -inf
[LightGBM] [Warning] No further splits with positive gain, best gain: -inf
[LightGBM] [Warning] No further splits with positive gain, best gain: -inf
[LightGBM] [Warning] No further splits with positive gain, best gain: -inf
[LightGBM] [Warning] No further splits with positive gain, best gain: -inf
[LightGBM] [Warning] No further splits with positive gain, best gain: -inf
[LightGBM] [Warning] No further splits with positive gain, best gain: -inf
[LightGBM] [Warning] No further splits with positive gain, best gain: -inf
[LightGBM] [Warning] No further splits with positive gain, best gain: -inf
[LightGBM] [Warning] No further splits with positive gain, best gain: -inf
[LightGBM] [Warning] No further splits with positive gain, best gain: -inf
[LightGBM] [Warning] No further splits with positive gain, best gain: -inf
[LightGBM] [Warning] No further splits with positive gain, best gain: -inf
[LightGBM] [Warning] No further splits with positive gain, best gain: -inf
[LightGBM] [Warning] No further splits with positive gain, best gain: -inf
[LightGBM] [Warning] No further splits with positive gain, best gain: -inf
[LightGBM] [Warning] No further splits with positive gain, best gain: -inf
[LightGBM] [Warning] No further splits with positive gain, best gain: -inf
[LightGBM] [Warning] No further splits with positive gain, best gain: -inf
[LightGBM] [Warning] No further splits with positive gain, best gain: -inf
[LightGBM] [Warning] No further splits with positive gain, best gain: -inf
[LightGBM] [Warning] No further splits with positive gain, best gain: -inf
[LightGBM] [Warning] No further splits with positive gain, best gain: -inf
[LightGBM] [Warning] No further splits with positive gain, best gain: -inf
[LightGBM] [Warning] No further splits with positive gain, best gain: -inf
[LightGBM] [Warning] No further splits with positive gain, best gain: -inf
[LightGBM] [Warning] No further splits with positive gain, best gain: -inf
[LightGBM] [Warning] No further splits with positive gain, best gain: -inf
[LightGBM] [Warning] No further splits with positive gain, best gain: -inf
[LightGBM] [Warning] No further splits with positive gain, best gain: -inf
[LightGBM] [Warning] No further splits with positive gain, best gain: -inf
[LightGBM] [Warning] No further splits with positive gain, best gain: -inf
[LightGBM] [Warning] No further splits with positive gain, best gain: -inf
[LightGBM] [Warning] No further splits with positive gain, best gain: -inf
[LightGBM] [Warning] No further splits with positive gain, best gain: -inf
[LightGBM] [Warning] No further splits with positive gain, best gain: -inf
[LightGBM] [Warning] No further splits with positive gain, best gain: -inf
[LightGBM] [Warning] No further splits with positive gain, best gain: -inf
[LightGBM] [Warning] No further splits with positive gain, best gain: -inf
[LightGBM] [Warning] No further splits with positive gain, best gain: -inf
[LightGBM] [Warning] No further splits with positive gain, best gain: -inf
[LightGBM] [Warning] No further splits with positive gain, best gain: -inf
[LightGBM] [Warning] No further splits with positive gain, best gain: -inf
[LightGBM] [Warning] No further splits with positive gain, best gain: -inf
[LightGBM] [Warning] No further splits with positive gain, best gain: -inf
[LightGBM] [Warning] No further splits with positive gain, best gain: -inf
[LightGBM] [Warning] No further splits with positive gain, best gain: -inf
[LightGBM] [Warning] No further splits with positive gain, best gain: -inf
[LightGBM] [Warning] No further splits with positive gain, best gain: -inf
[LightGBM] [Warning] No further splits with positive gain, best gain: -inf
[LightGBM] [Warning] No further splits with positive gain, best gain: -inf
[LightGBM] [Warning] No further splits with positive gain, best gain: -inf
[LightGBM] [Warning] No further splits with positive gain, best gain: -inf
[LightGBM] [Warning] Found whitespace in feature_names, replace with underlines
[LightGBM] [Info] Auto-choosing col-wise multi-threading, the overhead of testing was 0.000041 seconds.
You can set `force_col_wise=true` to remove the overhead.
[LightGBM] [Info] Total Bins 15
[LightGBM] [Info] Number of data points in the train set: 169, number of used features: 5
[LightGBM] [Info] Start training from score 3.319527
[LightGBM] [Warning] No further splits with positive gain, best gain: -inf
[LightGBM] [Warning] No further splits with positive gain, best gain: -inf
[LightGBM] [Warning] No further splits with positive gain, best gain: -inf
[LightGBM] [Warning] No further splits with positive gain, best gain: -inf
[LightGBM] [Warning] No further splits with positive gain, best gain: -inf
[LightGBM] [Warning] No further splits with positive gain, best gain: -inf
[LightGBM] [Warning] No further splits with positive gain, best gain: -inf
[LightGBM] [Warning] No further splits with positive gain, best gain: -inf
[LightGBM] [Warning] No further splits with positive gain, best gain: -inf
[LightGBM] [Warning] No further splits with positive gain, best gain: -inf
[LightGBM] [Warning] No further splits with positive gain, best gain: -inf
[LightGBM] [Warning] No further splits with positive gain, best gain: -inf
[LightGBM] [Warning] No further splits with positive gain, best gain: -inf
[LightGBM] [Warning] No further splits with positive gain, best gain: -inf
[LightGBM] [Warning] No further splits with positive gain, best gain: -inf
[LightGBM] [Warning] No further splits with positive gain, best gain: -inf
[LightGBM] [Warning] No further splits with positive gain, best gain: -inf
[LightGBM] [Warning] No further splits with positive gain, best gain: -inf
[LightGBM] [Warning] No further splits with positive gain, best gain: -inf
[LightGBM] [Warning] No further splits with positive gain, best gain: -inf
[LightGBM] [Warning] No further splits with positive gain, best gain: -inf
[LightGBM] [Warning] No further splits with positive gain, best gain: -inf
[LightGBM] [Warning] No further splits with positive gain, best gain: -inf
[LightGBM] [Warning] No further splits with positive gain, best gain: -inf
[LightGBM] [Warning] No further splits with positive gain, best gain: -inf
[LightGBM] [Warning] No further splits with positive gain, best gain: -inf
[LightGBM] [Warning] No further splits with positive gain, best gain: -inf
[LightGBM] [Warning] No further splits with positive gain, best gain: -inf
[LightGBM] [Warning] No further splits with positive gain, best gain: -inf
[LightGBM] [Warning] No further splits with positive gain, best gain: -inf
[LightGBM] [Warning] No further splits with positive gain, best gain: -inf
[LightGBM] [Warning] No further splits with positive gain, best gain: -inf
[LightGBM] [Warning] No further splits with positive gain, best gain: -inf
[LightGBM] [Warning] No further splits with positive gain, best gain: -inf
[LightGBM] [Warning] No further splits with positive gain, best gain: -inf
[LightGBM] [Warning] No further splits with positive gain, best gain: -inf
[LightGBM] [Warning] No further splits with positive gain, best gain: -inf
[LightGBM] [Warning] No further splits with positive gain, best gain: -inf
[LightGBM] [Warning] No further splits with positive gain, best gain: -inf
[LightGBM] [Warning] No further splits with positive gain, best gain: -inf
[LightGBM] [Warning] No further splits with positive gain, best gain: -inf
[LightGBM] [Warning] No further splits with positive gain, best gain: -inf
[LightGBM] [Warning] No further splits with positive gain, best gain: -inf
[LightGBM] [Warning] No further splits with positive gain, best gain: -inf
[LightGBM] [Warning] No further splits with positive gain, best gain: -inf
[LightGBM] [Warning] No further splits with positive gain, best gain: -inf
[LightGBM] [Warning] No further splits with positive gain, best gain: -inf
[LightGBM] [Warning] No further splits with positive gain, best gain: -inf
[LightGBM] [Warning] No further splits with positive gain, best gain: -inf
[LightGBM] [Warning] No further splits with positive gain, best gain: -inf
[LightGBM] [Warning] No further splits with positive gain, best gain: -inf
[LightGBM] [Warning] No further splits with positive gain, best gain: -inf
[LightGBM] [Warning] No further splits with positive gain, best gain: -inf
[LightGBM] [Warning] No further splits with positive gain, best gain: -inf
[LightGBM] [Warning] No further splits with positive gain, best gain: -inf
[LightGBM] [Warning] No further splits with positive gain, best gain: -inf
[LightGBM] [Warning] No further splits with positive gain, best gain: -inf
[LightGBM] [Warning] No further splits with positive gain, best gain: -inf
[LightGBM] [Warning] No further splits with positive gain, best gain: -inf
[LightGBM] [Warning] No further splits with positive gain, best gain: -inf
[LightGBM] [Warning] No further splits with positive gain, best gain: -inf
[LightGBM] [Warning] No further splits with positive gain, best gain: -inf
[LightGBM] [Warning] No further splits with positive gain, best gain: -inf
[LightGBM] [Warning] No further splits with positive gain, best gain: -inf
[LightGBM] [Warning] No further splits with positive gain, best gain: -inf
[LightGBM] [Warning] No further splits with positive gain, best gain: -inf
[LightGBM] [Warning] No further splits with positive gain, best gain: -inf
[LightGBM] [Warning] No further splits with positive gain, best gain: -inf
[LightGBM] [Warning] No further splits with positive gain, best gain: -inf
[LightGBM] [Warning] No further splits with positive gain, best gain: -inf
[LightGBM] [Warning] No further splits with positive gain, best gain: -inf
[LightGBM] [Warning] No further splits with positive gain, best gain: -inf
[LightGBM] [Warning] No further splits with positive gain, best gain: -inf
[LightGBM] [Warning] No further splits with positive gain, best gain: -inf
[LightGBM] [Warning] No further splits with positive gain, best gain: -inf
[LightGBM] [Warning] No further splits with positive gain, best gain: -inf
[LightGBM] [Warning] No further splits with positive gain, best gain: -inf
[LightGBM] [Warning] No further splits with positive gain, best gain: -inf
[LightGBM] [Warning] No further splits with positive gain, best gain: -inf
[LightGBM] [Warning] No further splits with positive gain, best gain: -inf
[LightGBM] [Warning] No further splits with positive gain, best gain: -inf
[LightGBM] [Warning] No further splits with positive gain, best gain: -inf
[LightGBM] [Warning] No further splits with positive gain, best gain: -inf
[LightGBM] [Warning] No further splits with positive gain, best gain: -inf
[LightGBM] [Warning] No further splits with positive gain, best gain: -inf
[LightGBM] [Warning] No further splits with positive gain, best gain: -inf
[LightGBM] [Warning] No further splits with positive gain, best gain: -inf
[LightGBM] [Warning] No further splits with positive gain, best gain: -inf
[LightGBM] [Warning] No further splits with positive gain, best gain: -inf
[LightGBM] [Warning] No further splits with positive gain, best gain: -inf
[LightGBM] [Warning] No further splits with positive gain, best gain: -inf
[LightGBM] [Warning] No further splits with positive gain, best gain: -inf
[LightGBM] [Warning] No further splits with positive gain, best gain: -inf
[LightGBM] [Warning] No further splits with positive gain, best gain: -inf
[LightGBM] [Warning] No further splits with positive gain, best gain: -inf
[LightGBM] [Warning] No further splits with positive gain, best gain: -inf
[LightGBM] [Warning] No further splits with positive gain, best gain: -inf
[LightGBM] [Warning] No further splits with positive gain, best gain: -inf
[LightGBM] [Warning] No further splits with positive gain, best gain: -inf
[LightGBM] [Warning] No further splits with positive gain, best gain: -inf
[LightGBM] [Warning] Found whitespace in feature_names, replace with underlines
[LightGBM] [Info] Auto-choosing col-wise multi-threading, the overhead of testing was 0.000025 seconds.
You can set `force_col_wise=true` to remove the overhead.
[LightGBM] [Info] Total Bins 11
[LightGBM] [Info] Number of data points in the train set: 83, number of used features: 3
[LightGBM] [Info] Start training from score 2.951807
[LightGBM] [Warning] No further splits with positive gain, best gain: -inf
[LightGBM] [Warning] No further splits with positive gain, best gain: -inf
[LightGBM] [Warning] No further splits with positive gain, best gain: -inf
[LightGBM] [Warning] No further splits with positive gain, best gain: -inf
[LightGBM] [Warning] No further splits with positive gain, best gain: -inf
[LightGBM] [Warning] No further splits with positive gain, best gain: -inf
[LightGBM] [Warning] No further splits with positive gain, best gain: -inf
[LightGBM] [Warning] No further splits with positive gain, best gain: -inf
[LightGBM] [Warning] No further splits with positive gain, best gain: -inf
[LightGBM] [Warning] No further splits with positive gain, best gain: -inf
[LightGBM] [Warning] No further splits with positive gain, best gain: -inf
[LightGBM] [Warning] No further splits with positive gain, best gain: -inf
[LightGBM] [Warning] No further splits with positive gain, best gain: -inf
[LightGBM] [Warning] No further splits with positive gain, best gain: -inf
[LightGBM] [Warning] No further splits with positive gain, best gain: -inf
[LightGBM] [Warning] No further splits with positive gain, best gain: -inf
[LightGBM] [Warning] No further splits with positive gain, best gain: -inf
[LightGBM] [Warning] No further splits with positive gain, best gain: -inf
[LightGBM] [Warning] No further splits with positive gain, best gain: -inf
[LightGBM] [Warning] No further splits with positive gain, best gain: -inf
[LightGBM] [Warning] No further splits with positive gain, best gain: -inf
[LightGBM] [Warning] No further splits with positive gain, best gain: -inf
[LightGBM] [Warning] No further splits with positive gain, best gain: -inf
[LightGBM] [Warning] No further splits with positive gain, best gain: -inf
[LightGBM] [Warning] No further splits with positive gain, best gain: -inf
[LightGBM] [Warning] No further splits with positive gain, best gain: -inf
[LightGBM] [Warning] No further splits with positive gain, best gain: -inf
[LightGBM] [Warning] No further splits with positive gain, best gain: -inf
[LightGBM] [Warning] No further splits with positive gain, best gain: -inf
[LightGBM] [Warning] No further splits with positive gain, best gain: -inf
[LightGBM] [Warning] No further splits with positive gain, best gain: -inf
[LightGBM] [Warning] No further splits with positive gain, best gain: -inf
[LightGBM] [Warning] No further splits with positive gain, best gain: -inf
[LightGBM] [Warning] No further splits with positive gain, best gain: -inf
[LightGBM] [Warning] No further splits with positive gain, best gain: -inf
[LightGBM] [Warning] No further splits with positive gain, best gain: -inf
[LightGBM] [Warning] No further splits with positive gain, best gain: -inf
[LightGBM] [Warning] No further splits with positive gain, best gain: -inf
[LightGBM] [Warning] No further splits with positive gain, best gain: -inf
[LightGBM] [Warning] No further splits with positive gain, best gain: -inf
[LightGBM] [Warning] No further splits with positive gain, best gain: -inf
[LightGBM] [Warning] No further splits with positive gain, best gain: -inf
[LightGBM] [Warning] No further splits with positive gain, best gain: -inf
[LightGBM] [Warning] No further splits with positive gain, best gain: -inf
[LightGBM] [Warning] No further splits with positive gain, best gain: -inf
[LightGBM] [Warning] No further splits with positive gain, best gain: -inf
[LightGBM] [Warning] No further splits with positive gain, best gain: -inf
[LightGBM] [Warning] No further splits with positive gain, best gain: -inf
[LightGBM] [Warning] No further splits with positive gain, best gain: -inf
[LightGBM] [Warning] No further splits with positive gain, best gain: -inf
[LightGBM] [Warning] No further splits with positive gain, best gain: -inf
[LightGBM] [Warning] No further splits with positive gain, best gain: -inf
[LightGBM] [Warning] No further splits with positive gain, best gain: -inf
[LightGBM] [Warning] No further splits with positive gain, best gain: -inf
[LightGBM] [Warning] No further splits with positive gain, best gain: -inf
[LightGBM] [Warning] No further splits with positive gain, best gain: -inf
[LightGBM] [Warning] No further splits with positive gain, best gain: -inf
[LightGBM] [Warning] No further splits with positive gain, best gain: -inf
[LightGBM] [Warning] No further splits with positive gain, best gain: -inf
[LightGBM] [Warning] No further splits with positive gain, best gain: -inf
[LightGBM] [Warning] No further splits with positive gain, best gain: -inf
[LightGBM] [Warning] No further splits with positive gain, best gain: -inf
[LightGBM] [Warning] No further splits with positive gain, best gain: -inf
[LightGBM] [Warning] No further splits with positive gain, best gain: -inf
[LightGBM] [Warning] No further splits with positive gain, best gain: -inf
[LightGBM] [Warning] No further splits with positive gain, best gain: -inf
[LightGBM] [Warning] No further splits with positive gain, best gain: -inf
[LightGBM] [Warning] No further splits with positive gain, best gain: -inf
[LightGBM] [Warning] No further splits with positive gain, best gain: -inf
[LightGBM] [Warning] No further splits with positive gain, best gain: -inf
[LightGBM] [Warning] No further splits with positive gain, best gain: -inf
[LightGBM] [Warning] No further splits with positive gain, best gain: -inf
[LightGBM] [Warning] No further splits with positive gain, best gain: -inf
[LightGBM] [Warning] No further splits with positive gain, best gain: -inf
[LightGBM] [Warning] No further splits with positive gain, best gain: -inf
[LightGBM] [Warning] No further splits with positive gain, best gain: -inf
[LightGBM] [Warning] No further splits with positive gain, best gain: -inf
[LightGBM] [Warning] No further splits with positive gain, best gain: -inf
[LightGBM] [Warning] No further splits with positive gain, best gain: -inf
[LightGBM] [Warning] No further splits with positive gain, best gain: -inf
[LightGBM] [Warning] No further splits with positive gain, best gain: -inf
[LightGBM] [Warning] No further splits with positive gain, best gain: -inf
[LightGBM] [Warning] No further splits with positive gain, best gain: -inf
[LightGBM] [Warning] No further splits with positive gain, best gain: -inf
[LightGBM] [Warning] No further splits with positive gain, best gain: -inf
[LightGBM] [Warning] No further splits with positive gain, best gain: -inf
[LightGBM] [Warning] No further splits with positive gain, best gain: -inf
[LightGBM] [Warning] No further splits with positive gain, best gain: -inf
[LightGBM] [Warning] No further splits with positive gain, best gain: -inf
[LightGBM] [Warning] No further splits with positive gain, best gain: -inf
[LightGBM] [Warning] No further splits with positive gain, best gain: -inf
[LightGBM] [Warning] No further splits with positive gain, best gain: -inf
[LightGBM] [Warning] No further splits with positive gain, best gain: -inf
[LightGBM] [Warning] No further splits with positive gain, best gain: -inf
[LightGBM] [Warning] No further splits with positive gain, best gain: -inf
[LightGBM] [Warning] No further splits with positive gain, best gain: -inf
[LightGBM] [Warning] No further splits with positive gain, best gain: -inf
[LightGBM] [Warning] No further splits with positive gain, best gain: -inf
[LightGBM] [Warning] No further splits with positive gain, best gain: -inf
[LightGBM] [Warning] No further splits with positive gain, best gain: -inf
# record thev estimated expected reward for each movie genere, under each possible combination of state variable
age_range = np.linspace(min(data_CEL_sample['age']),max(data_CEL_sample['age']),int(max(data_CEL_sample['age'])-min(data_CEL_sample['age'])+1)).astype(int)
import itertools
gender = np.array([0,1])
occupation_college = np.array([0,1])
occupation_executive = np.array([0,1])
occupation_other = np.array([0,1])
occupation_technician = np.array([0,1])
# result contains all possible combinations.
combinations = pd.DataFrame(itertools.product(age_range,gender,occupation_college,
occupation_executive,occupation_other,occupation_technician))
combinations.columns =['age','gender','occupation_college', 'occupation_executive','occupation_other','occupation_technician']
models_CEL['Comedy'].predict(combinations)
#models_CEL['Comedy'].predict(data_CEL_sample.iloc[np.where(data_CEL_sample['Comedy']==1)[0],userinfo_index])
array([3.1224, 3.0439, 3.7664, ..., 3.5822, 3.6663, 3.6364])
values = np.zeros((5,1312))
i=0
for movie_genere in movie_generes:
values[i,:] = models_CEL[movie_genere].predict(combinations)
i=i+1
#print(values)
result_CEL_nonlinear = combinations.copy()
i=0
for movie_genere in movie_generes:
#values = models_CEL[movie_genere].predict(combinations)
result_CEL_nonlinear.insert(len(result_CEL_nonlinear.columns), movie_genere, values[i,:])
i=i+1
result_CEL_nonlinear
age | gender | occupation_college | occupation_executive | occupation_other | occupation_technician | Comedy | Drama | Action | Thriller | Sci-Fi | |
---|---|---|---|---|---|---|---|---|---|---|---|
0 | 16 | 0 | 0 | 0 | 0 | 0 | 3.122379 | 3.576471 | 3.066448 | 3.583382 | 3.133766 |
1 | 16 | 0 | 0 | 0 | 0 | 1 | 3.043862 | 3.205939 | 3.232727 | 3.583382 | 3.133766 |
2 | 16 | 0 | 0 | 0 | 1 | 0 | 3.766441 | 3.910281 | 3.336623 | 3.717603 | 3.160268 |
3 | 16 | 0 | 0 | 0 | 1 | 1 | 3.687924 | 3.331463 | 3.345233 | 3.717603 | 3.160268 |
4 | 16 | 0 | 0 | 1 | 0 | 0 | 3.377340 | 3.649888 | 3.039056 | 3.923635 | 3.133766 |
... | ... | ... | ... | ... | ... | ... | ... | ... | ... | ... | ... |
1307 | 56 | 1 | 1 | 0 | 1 | 1 | 3.297553 | 3.090110 | 3.024221 | 3.658442 | 3.151436 |
1308 | 56 | 1 | 1 | 1 | 0 | 0 | 3.612166 | 3.695911 | 3.608458 | 3.740830 | 3.151436 |
1309 | 56 | 1 | 1 | 1 | 0 | 1 | 3.582210 | 3.165707 | 3.552889 | 3.740830 | 3.151436 |
1310 | 56 | 1 | 1 | 1 | 1 | 0 | 3.666311 | 3.283311 | 3.129195 | 3.740830 | 3.151436 |
1311 | 56 | 1 | 1 | 1 | 1 | 1 | 3.636355 | 3.103647 | 3.115987 | 3.740830 | 3.151436 |
1312 rows × 11 columns
# save the result to
result_CEL_nonlinear.to_csv('result_CEL_nonlinear.csv')
# read the result file
result_CEL_nonlinear = pd.read_csv('result_CEL_nonlinear.csv')
result_CEL_nonlinear = result_CEL_nonlinear.drop(result_CEL_nonlinear.columns[0], axis=1)
result_CEL_nonlinear
age | gender | occupation_college | occupation_executive | occupation_other | occupation_technician | Comedy | Drama | Action | Thriller | Sci-Fi | |
---|---|---|---|---|---|---|---|---|---|---|---|
0 | 16 | 0 | 0 | 0 | 0 | 0 | 3.122379 | 3.576471 | 3.066448 | 3.583382 | 3.133766 |
1 | 16 | 0 | 0 | 0 | 0 | 1 | 3.043862 | 3.205939 | 3.232727 | 3.583382 | 3.133766 |
2 | 16 | 0 | 0 | 0 | 1 | 0 | 3.766441 | 3.910281 | 3.336623 | 3.717603 | 3.160268 |
3 | 16 | 0 | 0 | 0 | 1 | 1 | 3.687924 | 3.331463 | 3.345233 | 3.717603 | 3.160268 |
4 | 16 | 0 | 0 | 1 | 0 | 0 | 3.377340 | 3.649888 | 3.039056 | 3.923635 | 3.133766 |
... | ... | ... | ... | ... | ... | ... | ... | ... | ... | ... | ... |
1307 | 56 | 1 | 1 | 0 | 1 | 1 | 3.297553 | 3.090110 | 3.024221 | 3.658442 | 3.151436 |
1308 | 56 | 1 | 1 | 1 | 0 | 0 | 3.612166 | 3.695911 | 3.608458 | 3.740830 | 3.151436 |
1309 | 56 | 1 | 1 | 1 | 0 | 1 | 3.582210 | 3.165707 | 3.552889 | 3.740830 | 3.151436 |
1310 | 56 | 1 | 1 | 1 | 1 | 0 | 3.666311 | 3.283311 | 3.129195 | 3.740830 | 3.151436 |
1311 | 56 | 1 | 1 | 1 | 1 | 1 | 3.636355 | 3.103647 | 3.115987 | 3.740830 | 3.151436 |
1312 rows × 11 columns
Analysis#
# calculate the expected reward of Comedy for female
TE_female=result_CEL_nonlinear.iloc[np.where(result_CEL_nonlinear['gender']==0)[0],6:11]/(41*(2**4))
TE_female=pd.DataFrame(TE_female.sum(axis=0))
TE_female.columns =['Expected Rating']
TE_female
Expected Rating | |
---|---|
Comedy | 3.500268 |
Drama | 3.309777 |
Action | 3.562432 |
Thriller | 3.605472 |
Sci-Fi | 2.960134 |
# calculate the expected reward of Comedy for female
TE_male=result_CEL_nonlinear.iloc[np.where(result_CEL_nonlinear['gender']==1)[0],6:11]/(41*(2**4))
TE_male=pd.DataFrame(TE_male.sum(axis=0))
TE_male.columns =['Expected Rating']
TE_male
Expected Rating | |
---|---|
Comedy | 3.365749 |
Drama | 3.321332 |
Action | 3.256846 |
Thriller | 3.447365 |
Sci-Fi | 2.960134 |
Conclusion: Among these five selected movie generes, Comedy
is the most popular one that received the highest expected rating. On the contrary, the estimated rating for Schi-Fi
is the lowest, both for males and females. In addition, the expected ratings of women are generally slightly higher than men, except for Drama
movies where men’s rating is expected to be 0.02/5 points higher than women.
linear model fitting#
models_CEL_linear = {}
# initialize the models we'll fit in Causal Effect Learning
for i in movie_generes:
models_CEL_linear[i] = None
from sklearn.linear_model import LinearRegression
for movie_genere in movie_generes:
models_CEL_linear[movie_genere] = LinearRegression()
models_CEL_linear[movie_genere].fit(data_CEL_sample.iloc[np.where(data_CEL_sample[movie_genere]==1)[0],userinfo_index],data_CEL_sample.iloc[np.where(data_CEL_sample[movie_genere]==1)[0],2] )
import itertools
gender = np.array([0,1])
occupation_college = np.array([0,1])
occupation_executive = np.array([0,1])
occupation_other = np.array([0,1])
occupation_technician = np.array([0,1])
# result contains all possible combinations.
combinations = pd.DataFrame(itertools.product(age_range,gender,occupation_college,
occupation_executive,occupation_other,occupation_technician))
combinations.columns =['age','gender','occupation_college', 'occupation_executive','occupation_other','occupation_technician']
values = np.zeros((5,1312))
i=0
for movie_genere in movie_generes:
values[i,:] = models_CEL_linear[movie_genere].predict(combinations)
i=i+1
#print(values)
result_CEL_linear = combinations
i=0
for movie_genere in movie_generes:
#values = models_CEL[movie_genere].predict(combinations)
result_CEL_linear.insert(len(result_CEL_linear.columns), movie_genere, values[i,:])
i=i+1
# the result is saved to
result_CEL_linear.to_csv('result_CEL_linear.csv')
# read the result file
result_CEL_linear = pd.read_csv('result_CEL_linear.csv')
result_CEL_linear = result_CEL_linear.drop(result_CEL_linear.columns[0], axis=1)
result_CEL_linear
age | gender | occupation_college | occupation_executive | occupation_other | occupation_technician | Comedy | Drama | Action | Thriller | Sci-Fi | |
---|---|---|---|---|---|---|---|---|---|---|---|
0 | 16 | 0 | 0 | 0 | 0 | 0 | 3.323169 | 3.453650 | 3.692167 | 3.482883 | 3.357668 |
1 | 16 | 0 | 0 | 0 | 0 | 1 | 3.325782 | 3.098945 | 3.628177 | 3.145705 | 3.886814 |
2 | 16 | 0 | 0 | 0 | 1 | 0 | 3.578237 | 3.666234 | 3.464250 | 3.392727 | 3.691633 |
3 | 16 | 0 | 0 | 0 | 1 | 1 | 3.580850 | 3.311529 | 3.400261 | 3.055549 | 4.220779 |
4 | 16 | 0 | 0 | 1 | 0 | 0 | 3.530090 | 3.566701 | 3.455929 | 3.741310 | 3.570002 |
... | ... | ... | ... | ... | ... | ... | ... | ... | ... | ... | ... |
1307 | 56 | 1 | 1 | 0 | 1 | 1 | 3.494923 | 3.259652 | 2.899359 | 3.006980 | 2.469963 |
1308 | 56 | 1 | 1 | 1 | 0 | 0 | 3.444164 | 3.514824 | 2.955027 | 3.692742 | 1.819186 |
1309 | 56 | 1 | 1 | 1 | 0 | 1 | 3.446776 | 3.160120 | 2.891038 | 3.355564 | 2.348332 |
1310 | 56 | 1 | 1 | 1 | 1 | 0 | 3.699231 | 3.727408 | 2.727111 | 3.602585 | 2.153151 |
1311 | 56 | 1 | 1 | 1 | 1 | 1 | 3.701844 | 3.372704 | 2.663122 | 3.265407 | 2.682297 |
1312 rows × 11 columns
Analysis#
# calculate the expected reward of Comedy for female
TE_female_linear=result_CEL_linear.iloc[np.where(result_CEL_linear['gender']==0)[0],6:11]/(41*(2**4))
TE_female_linear=pd.DataFrame(TE_female_linear.sum(axis=0))
TE_female_linear.columns =['Expected Rating']
TE_female_linear
Expected Rating | |
---|---|
Comedy | 3.579924 |
Drama | 3.402675 |
Action | 3.282099 |
Thriller | 3.511989 |
Sci-Fi | 3.082199 |
# calculate the expected reward of Comedy for female
TE_male_linear=result_CEL_linear.iloc[np.where(result_CEL_linear['gender']==1)[0],6:11]/(41*(2**4))
TE_male_linear=pd.DataFrame(TE_male_linear.sum(axis=0))
TE_male_linear.columns =['Expected Rating']
TE_male_linear
Expected Rating | |
---|---|
Comedy | 3.445089 |
Drama | 3.423679 |
Action | 3.073189 |
Thriller | 3.236301 |
Sci-Fi | 2.957766 |
Conclusion: The expected ratings obtained under linear model is generally consistent with the result under nonlinear model.
Online Learning#
In this section, we aim to implement the contextual TS to learn the optimal policy online. Specifically, we assume that, for each arm \(i\), $\(R_t(i)\sim \mathcal{N}(\boldsymbol{s}_i^T \boldsymbol{\gamma},\sigma^2).\)$
import pandas as pd
import numpy as np
from sklearn.linear_model import LinearRegression
from causaldm.learners.CPL4.CMAB import _env_realCMAB as _env
from causaldm.learners.CPL4.CMAB import LinTS
env = _env.Single_Contextual_Env(seed = 0, Binary = False)
K = env.K
p = env.p
logged_data, arms = env.get_logged_dat()
CEL_results = pd.read_csv('result_CEL_nonlinear.csv').iloc[:,1:]
Estimated \(\sigma\) and \(\boldsymbol{\gamma}\)#
Here, we estimated the \(\sigma\) and \(\boldsymbol{\gamma}\) based on the logged data and the estimated results obtained from the causal effect learning (CEL) step.
mean_error = []
for genere in arms:
genere_dat = logged_data[genere][['age','gender_M',
'occupation_college/grad student', 'occupation_executive/managerial',
'occupation_other', 'occupation_technician/engineer','rating']]
model = LinearRegression().fit(CEL_results.iloc[:,:-5], CEL_results[genere])
genere_error = genere_dat.rating.to_numpy() - model.predict(np.array(genere_dat.iloc[:,:-1]))
mean_error += genere_error.tolist()
sigma = np.std(mean_error,ddof=1)
gamma = []
for genere in arms:
model = LinearRegression().fit(CEL_results.iloc[:,:-5], CEL_results[genere])
gamma+=[model.intercept_] + list(model.coef_)
Run Informative TS#
Here, we run an informative TS with informative prior information, including the estimated \(\sigma\) and \(\gamma\). Specifically, we use \(\mathcal{N}(\hat{\boldsymbol{\gamma}},.05I)\) as the prior distribution of \(\gamma\). In total, we ran 50 replicates, each with 5000 total steps, to get the expected performance of online learning.
T = 20000
S = 50
sigma1 = .3
cum_reward_informative = []
for seed in range(S):
env = _env.Single_Contextual_Env(seed = seed, Binary = False)
prior_theta_u = np.array(gamma)
prior_theta_cov = sigma1*np.identity(p)
informative_TS= LinTS.LinTS_Gaussian(sigma = sigma, prior_theta_u = prior_theta_u,
prior_theta_cov = prior_theta_cov,
K = K, p = p,seed = seed)
cum_reward_informative_t = []
rec_action_informative_t = []
for t in range(T):
X, feature_info= env.get_Phi(t)
A = informative_TS.take_action(X)
R = env.get_reward(t,A)
informative_TS.receive_reward(t,A,R,X)
cum_reward_informative_t.append(R)
rec_action_informative_t.append(A)
cum_reward_informative_t = np.cumsum(cum_reward_informative_t)/(np.array(range(T))+1)
cum_reward_informative.append(cum_reward_informative_t)
Run Uninformative TS#
To further show the advantages of integrating the information from a CEL step, we run an uninformative TS with uninformative prior information. Specifically, we use \(\mathcal{N}(\boldsymbol{0},1000I)\) as the prior distribution of \(\gamma\). In total, we ran 50 replicates, each with 5000 total steps, to get the expected performance of online learning.
T = 20000
S = 50
cum_reward_uninformative = []
for seed in range(S):
env = _env.Single_Contextual_Env(seed = seed, Binary = False)
K = env.K
p = env.p
prior_theta_u = np.zeros(p)
prior_theta_cov = 1000*np.identity(p)
uninformative_TS= LinTS.LinTS_Gaussian(sigma = sigma, prior_theta_u = prior_theta_u,
prior_theta_cov = prior_theta_cov,
K = K, p = p,seed = seed)
cum_reward_uninformative_t = []
rec_action_uninformative_t = []
for t in range(T):
X, feature_info = env.get_Phi(t)
A = uninformative_TS.take_action(X)
R = env.get_reward(t,A)
uninformative_TS.receive_reward(t,A,R,X)
cum_reward_uninformative_t.append(R)
rec_action_uninformative_t.append(A)
cum_reward_uninformative_t = np.cumsum(cum_reward_uninformative_t)/(np.array(range(T))+1)
cum_reward_uninformative.append(cum_reward_uninformative_t)
Run Personalized Greedy#
We also run a greedy algorithm using the results of the CEL-HTE step as a natural baseline. In particular, for each round, we estimated the expected reward for each arm solely based on the estimated results from the CEL step and then pulled the arm with the highest expected reward.
T = 20000
S = 50
cum_reward_greedy = []
for seed in range(S):
env = _env.Single_Contextual_Env(seed = seed, Binary = False)
cum_reward_greedy_t = []
rec_action_greedy_t = []
for t in range(T):
X, feature_info= env.get_Phi(t)
A = np.argmax(X.dot(np.array(gamma)))
R = env.get_reward(t,A)
cum_reward_greedy_t.append(R)
rec_action_greedy_t.append(A)
cum_reward_greedy_t = np.cumsum(cum_reward_greedy_t)/(np.array(range(T))+1)
cum_reward_greedy.append(cum_reward_greedy_t)
Run Naive Greedy#
We also run a greedy algorithm on the CEL-ATE results, which serve as another natural baseline. For each round, we recommend thrillers to the user, as it has the highest expected average reward for both male and female users.
T = 20000
S = 50
cum_reward_nvgreedy = []
for seed in range(S):
env = _env.Single_Contextual_Env(seed = seed, Binary = False)
cum_reward_nvgreedy_t = []
rec_action_nvgreedy_t = []
for t in range(T):
A = 3
R = env.get_reward(t,A)
cum_reward_nvgreedy_t.append(R)
rec_action_nvgreedy_t.append(A)
cum_reward_nvgreedy_t = np.cumsum(cum_reward_nvgreedy_t)/(np.array(range(T))+1)
cum_reward_nvgreedy.append(cum_reward_nvgreedy_t)
Results#
On the one hand, while the greedy algorithm outperforms the TS algorithms in the early stages due to extra exploration, both TS algorithms continue to learn new information from the environment and eventually outperform the greedy algorithm. On the other hand, when the results of the uninformative TS are compared to the results of the informative TS, it is clear that the TS algorithm with an informative prior outperforms the uninformative TS, especially in the early stages, due to the use of prior information obtained from the CEL step. Based on the result of the last replicate of the informative TS, the mean of the final estimation of the posterior distribution of \(\boldsymbol{\gamma}\) is summarized as follows (it can be retrieved by informative_TS.u
):
intercept |
age |
gender |
college/grad student |
executive/managerial |
other |
technician/engineer |
|
---|---|---|---|---|---|---|---|
Comedy |
3.426 |
0.001 |
-0.045 |
-0.191 |
-0.062 |
-0.223 |
-0.149 |
Drama |
3.884 |
-0.010 |
-0.029 |
-0.161 |
-0.011 |
0.039 |
-0.117 |
Action |
2.429 |
0.023 |
-0.287 |
0.151 |
0.391 |
-0.391 |
-0.182 |
Thriller |
3.674 |
-0.008 |
-0.040 |
-0.267 |
-0.028 |
-0.000 |
-0.061 |
Sci-Fi |
3.114 |
0.004 |
-0.041 |
0.088 |
-.004 |
-0.063 |
-0.578 |
To use the estimated results greedily, we can calculate the mean reward of each movie genre using the estimated \(\boldsymbol{\gamma}\) and the incoming user’s information, and then recommend the genre with the highest estimated mean reward.
import seaborn as sns
import matplotlib.pyplot as plt
result = pd.DataFrame({'rep':np.concatenate([np.ones(T)*rep for rep in range(S)]*4),
't':np.concatenate([range(T)]*4*S),
'Reward':np.concatenate(cum_reward_informative+cum_reward_uninformative+cum_reward_greedy+cum_reward_nvgreedy),
'Algo':['info_LinTS']*T*S+['uninfo_LinTS']*T*S+['personalized greedy']*T*S+['naive greedy']*T*S})
sns.lineplot(data=result[result.t>0], x='t', y="Reward", hue="Algo", ci = 95,
n_boot = 20, linewidth = 1.0, markers = False)
plt.legend(bbox_to_anchor=(1.02, 1), loc='upper left', borderaxespad=0)
plt.ylabel('Average Reward')
plt.ylim(3.35, 3.55)
plt.savefig('my_figure.png', bbox_inches='tight')
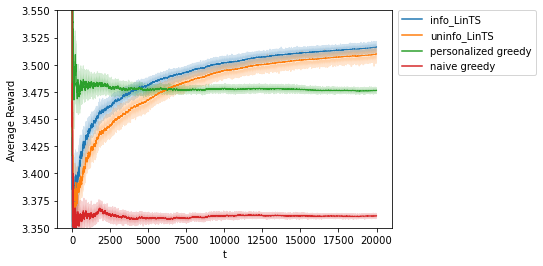